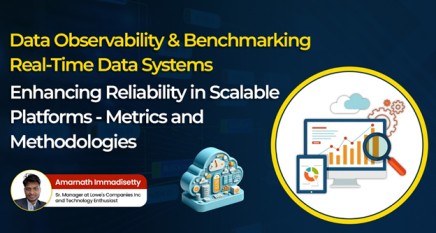
Data observability is becoming increasingly essential in today’s data-driven environments, particularly when it comes to ensuring the reliability and performance of real-time data systems. This article explores the intersection of data observability and benchmarking in scalable platforms, emphasizing metrics and methodologies that enhance data reliability.
Understanding Data Observability
Data observability refers to the comprehensive monitoring and analysis of data systems to ensure their health and performance. It encompasses a range of practices that allow organizations to detect anomalies, monitor data quality, and understand data flows throughout their pipelines. Unlike traditional data quality checks, which may occur at specific points in the data lifecycle, data observability provides continuous insights into the entire ecosystem, enabling proactive management of data-related issues.
Key Components of Data Observability
- Metrics: Metrics are fundamental to understanding the state of a data system. They provide quantitative insights into various aspects of data quality, such as freshness, accuracy, volume, and schema integrity.
- Logs: Logs offer detailed records of events within a system, helping teams trace back through actions taken on data and identify where issues may have occurred.
- Traces: Traces allow organizations to follow the path of a particular piece of data through its lifecycle, providing visibility into how it moves through different systems and transformations.
These components work together to create a holistic view of the data environment, enabling teams to quickly identify and resolve issues before they impact business operations.
Key Metrics for Benchmarking Real-Time Data Systems
Metric | Description | Importance |
Data Freshness | Measures how current the data is within the system. | Critical for real-time applications where outdated information can lead to poor decision-making. |
Data Volume | Refers to the amount of data being processed. | Helps manage storage needs and capacity planning effectively. |
Error Rates | Tracks how often issues occur within the system. | Allows teams to address root causes proactively, improving overall system reliability. |
Pipeline Latency | Measures the time it takes for data to move through various stages in a pipeline. | Reducing latency is crucial for applications that require immediate insights. |
Schema Changes | Monitors changes in schema to ensure compatibility across systems. | Helps maintain data integrity and prevents issues related to transformations. |
The Importance of Real-Time Monitoring
In an era where businesses rely heavily on real-time analytics for decision-making, the need for robust data observability practices is paramount. Real-time monitoring allows organizations to:
- Reduce Mean Time To Detect (MTTD): By continuously monitoring data pipelines, teams can quickly identify when something goes wrong.
- Minimize Mean Time To Resolve (MTTR): Swift detection leads to faster resolution times, reducing downtime and improving overall system reliability.
- Enhance Data Quality: Continuous oversight ensures that any anomalies or inconsistencies are addressed immediately, maintaining high standards for data integrity.
Benchmarking Real-Time Data Systems
Benchmarking is a vital practice that allows organizations to assess the performance of their data systems against established standards or metrics. When combined with data observability, benchmarking can significantly enhance the reliability of real-time systems.
Key Metrics for Benchmarking
- Data Freshness: Measures how current the data is within the system. Freshness is critical for real-time applications where outdated information can lead to poor decision-making.
- Data Volume: Refers to the amount of data being processed. Monitoring volume helps organizations manage their storage needs and capacity planning effectively.
- Error Rates: Tracking error rates helps identify how often issues occur within the system, allowing teams to address root causes proactively.
- Pipeline Latency: Measures the time it takes for data to move through various stages in a pipeline. Reducing latency is crucial for applications that require immediate insights.
- Schema Changes: Monitoring changes in schema helps ensure that all systems remain compatible and that transformations do not lead to data integrity issues.
Methodologies for Effective Benchmarking
To effectively benchmark real-time data systems, organizations should adopt several methodologies:
- Establish Baselines: Collect historical performance data to determine average values for key metrics over time. This provides a reference point for evaluating future performance.
- Set Thresholds: Define acceptable ranges for each metric based on historical baselines. Alerts should be configured to notify teams when metrics fall outside these thresholds.
- Conduct Regular Reviews: Periodically review benchmarking results against current performance metrics to identify trends, anomalies, or areas needing improvement.
- Utilize Automated Tools: Leverage machine learning models that can automatically adjust thresholds based on changing patterns in the data environment.
Methodologies for Effective Benchmarking
Methodology | Description |
Establish Baselines | Collect historical performance data to determine average values for key metrics over time. |
Set Thresholds | Define acceptable ranges for each metric based on historical baselines and configure alerts. |
Conduct Regular Reviews | Periodically review benchmarking results against current performance metrics to identify trends. |
Utilize Automated Tools | Leverage machine learning models that can automatically adjust thresholds based on changing patterns in the data environment. |
Challenges in Implementing Data Observability
While implementing robust data observability practices offers numerous benefits, organizations may face several challenges:
- Data Silos: Isolated datasets can hinder comprehensive monitoring efforts. Organizations must work towards integrating disparate systems for a unified view.
- Standardization Issues: Inconsistent formats across systems complicate monitoring efforts. Standardizing telemetry and logging practices is essential for effective observability.
- Storage Costs: Monitoring large volumes of data can lead to increased storage costs. Organizations need strategies to optimize storage while maintaining necessary observability capabilities.
- Collaborative Efforts: Successful implementation requires collaboration across various teams—data engineers, analysts, and IT personnel must work together towards common goals.
The Future of Data Observability
As technology continues to evolve, so too will the practices surrounding data observability:
- Increased Automation: Future tools will leverage advanced machine learning algorithms to automate anomaly detection and reporting processes.
- Greater Focus on Self-Service Analytics: Empowering users with self-service access will reduce dependencies on technical teams and speed up decision-making processes.
- Integration with AI/ML Solutions: The incorporation of AI and machine learning into observability tools will enhance predictive capabilities, allowing organizations to anticipate issues before they arise.
Conclusion
Data observability is essential for ensuring the reliability of real-time data systems in an increasingly complex digital landscape. By implementing robust monitoring practices and establishing clear benchmarking methodologies, organizations can enhance their ability to manage data effectively. As businesses continue to embrace advanced technologies and real-time analytics, prioritizing data observability will be crucial in maintaining high-quality standards and driving informed decision-making processes.
In summary, organizations must recognize the importance of integrating comprehensive observability practices into their operational frameworks. By doing so, they will not only improve their current capabilities but also position themselves for success in an ever-evolving technological landscape.
Amarnath Immadisetty is a seasoned technology leader with over 17 years of experience in software engineering. Currently serving as the Senior Manager of Software Engineering at Lowe’s, he oversees a team of more than 20 engineers. Amarnath is known for driving transformation through innovative solutions in customer data platforms, software development, and large-scale data analytics, significantly enhancing business performance.
Throughout his career, Amarnath has held key positions at notable companies such as Target, Uniqlo, and CMC Limited. His strong foundation in technical leadership and engineering excellence enables him to foster innovation in data-driven decision-making. Passionate about mentoring the next generation of engineers, Amarnath actively promotes diversity and inclusion within the tech industry, believing that diverse teams lead to better innovation and problem-solving.
Ad:
Transform your digital presence with HashAdv Branding. ( https://hashadv.in/ ). Offering expert graphic designing, digital marketing, and web development services to elevate your brand and drive impactful results.